畢業(yè)設(shè)計論文 外文文獻翻譯 中英文對照 快速和簡單 的溝通使用Co – SVM對圖像檢索進行主動學(xué)習(xí)
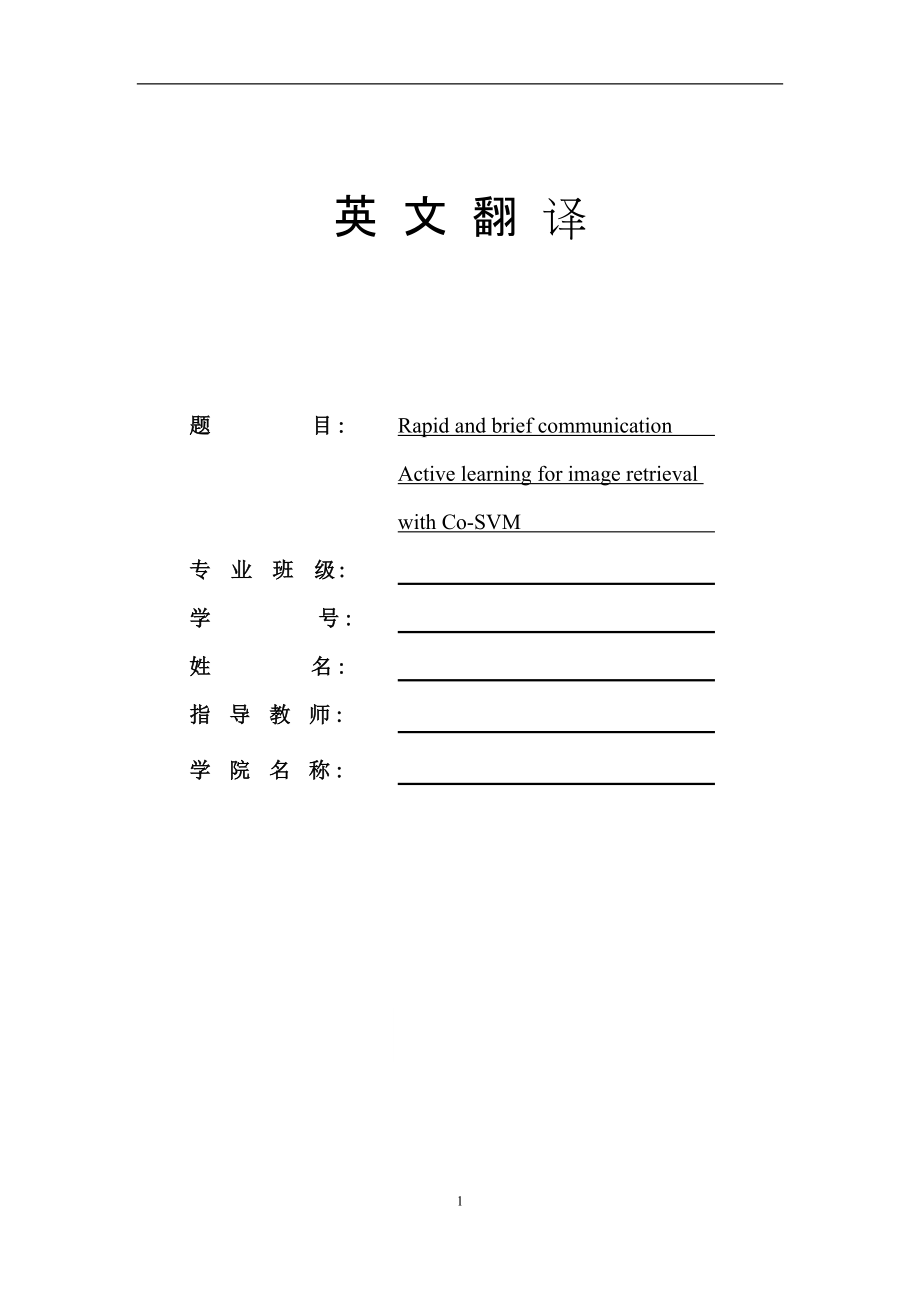


《畢業(yè)設(shè)計論文 外文文獻翻譯 中英文對照 快速和簡單 的溝通使用Co – SVM對圖像檢索進行主動學(xué)習(xí)》由會員分享,可在線閱讀,更多相關(guān)《畢業(yè)設(shè)計論文 外文文獻翻譯 中英文對照 快速和簡單 的溝通使用Co – SVM對圖像檢索進行主動學(xué)習(xí)(14頁珍藏版)》請在裝配圖網(wǎng)上搜索。
1、 英 文 翻 譯 題 目: Rapid and brief communication Active learning for image retrieval with Co-SVM 專 ?業(yè)? 班? 級: 學(xué) ?號: 姓 ?名: 指 導(dǎo) 教 師:
2、 學(xué) 院 名 稱: 13 快速和簡單的溝通使用Co – SVM對圖像檢索進行主動學(xué)習(xí) 摘 要 在相關(guān)反饋算法中,選擇性抽樣通常用來減少標簽成本以及探討未標記的數(shù)據(jù)。在本文中,為了提高圖像檢索選擇性抽樣的表現(xiàn),我們提出了一個積極的學(xué)習(xí)算法,這個算法被稱為Co – SVM。在Co – SVM算法中,色彩和紋理很自然的當(dāng)做一幅圖像足夠的、不相關(guān)的試圖。我們能夠分別在顏色和紋理的特征子空間上學(xué)習(xí)SVM分類器。因此,這兩個分類器被用于分類未標記的數(shù)據(jù)。當(dāng)
3、兩個分類器分辨出不同時,這些未標記的數(shù)據(jù)就成為了選擇標簽。實驗結(jié)果表明我們提出的這種算法對圖像檢索是非常有利的。 1 前 言 相關(guān)反饋是提高圖像檢索性能的一種重要的方法[1]。對于企業(yè)大型圖像數(shù)據(jù)庫檢索的問題,標記圖像總是比未標記的圖像罕見。當(dāng)只提供一個小的標記圖像時,如何利用大量未標記的圖像去增強學(xué)習(xí)算法的性能已成為一個熱門話題。Tong 和Chang提出了一種主動學(xué)習(xí)的算法,這種算法叫做SVMAct ive [2]。他們認為處于邊界的樣本是最豐富的。因此在每一輪相關(guān)反饋中,能作為標簽返回給用戶的是那些最接近支持矢量邊界的圖像。 通常情況下,圖像的特征表示是一個組合的,多樣化的功能,
4、如顏色,紋理,形狀等。例如,對于指定的圖像,不同特征的重要性是顯著不同的。另一方面,重要性相同的特征對于不同圖像也是不同的。例如,通常情況下,顏色是比形狀更為突出的圖像。然而,檢索結(jié)果是所有特征的平均作用,而忽略了個別特征鮮明的特性。一些研究顯示多視角的學(xué)習(xí)比單視圖的假說要好[3,4]。 在本文中,我們把顏色和紋理作為圖像描述的兩個充分的、不相關(guān)的圖像特征。受SVMAct ive的啟發(fā),我們提出了一種新的主動學(xué)習(xí)法,這種方法叫做Co–SVM。首先,在不同的特征表示上分別學(xué)習(xí)SVM分類器,然后這些分類器用來從未標記的數(shù)據(jù)中選擇最翔實的合作樣本,最后,這些翔實樣本將作為標簽返回給用戶。 2 支
5、持向量機 作為一個有效的二元分類器,將SVM用于圖像檢索相關(guān)反饋的分類是特別適合的[5]。隨著標簽圖像,SVM學(xué)習(xí)一個邊界(即超平面),就是從帶有最大利潤的不相關(guān)的圖片中分離相關(guān)圖像。處于邊界一側(cè)的圖像被認為是相關(guān)的,而處于另一側(cè)的則被認為是不相關(guān)的。給定一個標記圖像集(x1,y1), . . . ,(xn,yn) , xi 是一幅圖像的特征描述,yi ∈ {?1,+1} 是類標簽(- 1表示正極,+1表示負極)。訓(xùn)練SVM分類器會導(dǎo)致下面的二次優(yōu)化問題: S.t.: 其中C是一個常數(shù),k為內(nèi)核的功能。邊界(超平面)是 其中滿足任何支持向量的條件是: 該分類函數(shù)可以寫為:
6、3 合作支持向量機 3.1. 雙試圖計劃 假設(shè)圖像的顏色特征和紋理特征是兩個互不相關(guān)的觀點是自然的也是合理的。假設(shè)x={c1, . . . ,ci , t1 , . . . ,tj } 是一幅圖像的特征表示,其中 {c1, . . . ,ci}和 {t1, . . . ,tj} 分別是顏色屬性和紋理屬性。為簡單起見,我們定義特征表示空間V = VC VT , 而{c1, . . . ,ci}∈VC , {t1, . . . ,tj }∈VT。為了盡可能找到相關(guān)的圖像,像一般相關(guān)反饋的方法,在第一階段的聯(lián)合視圖V中支持向量機用于學(xué)習(xí)標記的樣本分類h。通過h未標記集被分為正面和負面的.然后m
7、的正面形象將返回到用戶的標簽。在第二個階段,通過VC顏色視圖和VT紋理視圖SVM用于在標記樣本上分別學(xué)習(xí)hc和ht兩種分類器 。對于兩個分類有分歧的未標記的樣本推薦給用戶做標簽,并將其命名為爭奪樣本。也就是說,爭論樣本以HC(CP)的分類劃分為陽性,以HT(TN)的分類劃分為陰性?;蛞訦C(CN)的分類劃分為陰性,以HT(TP)的分類劃分為陽性。對于每一個分類器,樣品之間的超平面(邊界)的距離可以被看作信心程度。越大的距離,越高的信任度。為了確保用戶可以標簽最翔實的樣本,在兩種意見上接近超平面的樣本被推薦給用戶作為標簽。 3.2. 多視圖計劃 在兩個個案中,提出的算法很容易擴展到多視圖計劃
8、。假設(shè),一個是彩色圖像特征被表示為V = V2的 V1的??? Vk,鉀> 2條所界定,每個VI,i= 1,鉀對應(yīng)的彩色圖有不同的看法。然后在每一個視圖上可以學(xué)習(xí)K向量機分類。所有未標記的數(shù)據(jù)被 k 支持向量機分類器歸類為陽性 (+ 1) 或陰性 (?1)。定義置信度D(x) ____ki=1sign(hi(x)) _____。置信度可以反映上一示例中指定的所有分類器的一致性。置信度越高,分類器越一致。相反,低置信度說明分類器是不確定的。這些不確定的樣本標簽將導(dǎo)致性能的最大改進。因此,其信任度是最低的未標記的樣本被視為爭奪樣本。 3.3. SVM 簡介 SVM( Support Vect
9、or machine, 支持向量機) 方法[4]是建立在統(tǒng)計學(xué)習(xí)理論的VC 維理論和結(jié)構(gòu)風(fēng)險最小原理基礎(chǔ)上的,根據(jù)有限的樣本信息在模型的復(fù)雜性和學(xué)習(xí)能力之間尋求最佳的折衷, 以期獲得最好的推廣能力。 SVM的主要思想是建立一個超平面作為決策曲面, 使得正例和反例之間的隔離邊緣被最大化。對于二維線性可分情況, 令H 為把兩類訓(xùn)練樣本沒有錯誤地分開的分類線, H1, H2分別為過各類中離分類線最近的樣本且平行于分類線的直線, 它們之間的距離叫做分類間隔。所謂最優(yōu)分類線就是要求分類線不但能將兩類正確分開, 而且使分類間隔最大。在高維空間, 最優(yōu)分類線就成為最優(yōu)分類面。 實驗: 為了驗證了在性能
10、上改進算法的有效性,我們將它與Tong & Chang SVMAct ive及傳統(tǒng)的相關(guān)反饋算法支持向量機進行比較。Corel 圖像光盤從所選子集中執(zhí)行實驗。在我們的子集中有 50 個類別。每個類別包含100個圖像,一共有5000個圖像。該類別有不同的語義,如動物,建筑,景觀等的含義。 我們的實驗的主要目的是驗證聯(lián)合支持向量機的學(xué)習(xí)機制是否有用,因此,我們只用來簡單的顏色和紋理特征來表示圖RGB 顏色特征包括 125 維顏色直方圖矢量和 6 維顏色矩矢量。像。紋理特征提取使用 3 級離散小波變換 (DWT)。均值和方差平均每10個子帶被排列成20維紋理特征矢量。在支持向量機分類器中采用徑向基
11、核。該內(nèi)核寬度是由交叉驗證的方法得到的。 每個類別500個圖像的前10 個形象被選擇作為查詢圖像來探測檢索性能。在每一輪中,只有前10名的圖像標記和10個最不自信的圖片集爭奪選定的標簽。以下文本中的所有精度都為平均測試的所有圖像的準確性。第三輪及第五輪相關(guān)反饋后2和3是描述3種算法的相關(guān)反饋后準確性的范圍曲線。從比較的結(jié)果中,我們可以看到擬議的算法 (聯(lián)合-支持向量機) 勝于 SVMAct (活動支持向量機) 和傳統(tǒng)的相關(guān)反饋方法 (支持向量機)。此外,我們在調(diào)查前100名中前10名各種算法的準確性并有五輪的反饋。由于空間有限我們只分別在圖片1和圖片2中表示了前 30 和前 50的結(jié)果。
12、 圖一 前30名的平均圖像檢索 圖二前30名的平均圖像檢索 5 相關(guān)作品: co-training [3] 和 co-testing [4]是兩種典型的多視點學(xué)習(xí)算法 。co-training 算法采用合作學(xué)習(xí)策略,要求這兩種視圖的數(shù)據(jù)是兼容和冗余的。我們曾嘗試結(jié)合 co-training增加顏色和紋理分類器的性能,但結(jié)果卻更糟??紤]到 co-training 的狀況,人們會很自然的發(fā)現(xiàn)顏色屬性和紋理屬性是一幅彩色圖像不兼容的并且不相關(guān)的屬性。相比之下,co-
13、testing 的要求圖像應(yīng)該是兼容并且相關(guān)的,使分類器能更獨立的分類。Tong和Chang首先推出的 SVMAct [2] 的是主動學(xué)習(xí)關(guān)于圖像檢索相關(guān)反饋的方法。他們認為在處于邊界的示例可以盡快減少版本空間,即消除假說。因此,每次的相關(guān)反饋,最接近該超平面的圖像會作為標記返回給用戶。SVMActive 是在單一視圖的情況下對版本空間最小化最佳的。建議的算法可以被認為是 SVMActive 在多個視圖的情況下的擴展。 6 總結(jié) 在這篇文章中,我們建議積極學(xué)習(xí)相關(guān)反饋中的選擇性的抽樣算法——聯(lián)合支持向量機。為了提高性能,相關(guān)反饋分為兩個階段。第一階段我們通過未標記的圖像的相似性查詢排名,并
14、讓用戶可以像常見的相關(guān)反饋算法那樣標簽頂部圖像。在第二階段,為了減少標簽規(guī)定,只有一組內(nèi)容最豐富的示例被聯(lián)合-支持向量機所選擇作為標簽。實驗結(jié)果顯示聯(lián)合-支持向量機與SVMActive和沒有主動學(xué)習(xí)的傳統(tǒng)相關(guān)反饋算法相比,有明顯的改善。 鳴謝,第一作者被授予諾基亞博士后獎學(xué)金。 參考資料 [1] Y. Rui, T.S. Huang, S.F. Chang, Image retrieval: current techniques, promising directions and open issues, J. Visual Commun. Image Representation
15、10 (1999) 39–62. [2] S. Tong, E. Chang, Support vector machine active learning for image retrieval, in: Proceedings of the Ninth ACM International Conference on Multimedia, 2001, pp. 107–118. [3] A. Blum, T. Mitchell, Combining labeled and unlabeled data with co-training, in: Proceedings of the
16、 11th Annual Conference on Computational Learning Theory, 1998, pp. 92–100. [4] I. Muslea, S. Minton, C.A. Knoblock, Selective sampling with redundant views, in: Proceedings of the 17th National Conference on Artificial Intelligence, 2000, pp. 621–626. [5] V. Vapnik, Statistical Learning Theory
17、, Wiley, New York, 1998. Rapid and brief communication Active learning for image retrieval with Co-SVM Abstract In relevance feedback algorithms, selective sampling is often used to reduce the cost of labeling and explore the unlabeled data. In this paper, we proposed an ac
18、tive learning algorithm, Co-SVM, to improve the performance of selective sampling in image retrieval. In Co-SVM algorithm, color and texture are naturally considered as sufficient and uncorrelated views of an image. SVM classifiers are learned in color and texture feature subspaces, respectively. Th
19、en the two classifiers are used to classify the unlabeled data. These unlabeled samples which are differently classified by the two classifiers are chose to label. The experimental results show that the proposed algorithm is beneficial to image retrieval. 1. Introduction Relevance feedback is an i
20、mportant approach to improve the performance of image retrieval systems [1]. For largescale image database retrieval problem, labeled images are always rare compared with unlabeled images. It has become a hot topic how to utilize the large amounts of unlabeled images to augment the performance of th
21、e learning algorithms when only a small set of labeled images is available. Tong and Chang proposed an active learning paradigm, named SVMAct ive [2]. They think that the samples lying beside the boundary are the most informative. Therefore, in each round of relevance feedback, the images that are c
22、losest to the support vector boundary are returned to users for labeling. Usually, the feature representation of an image is a combination of diverse features, such as color, texture, shape, etc. For a specified example, the contribution of different features is significantly different. On the oth
23、er hand, the importance of the same feature is also different for differentsamples. For example, color is often more prominent than shape for a landscape image. However, the retrieval results are the averaging effort of all features, which ignores the distinct properties of individual feature. Some
24、 works have suggested that multi-view learning can do much better than the single-view learning in eliminating the hypotheses consistent with the training set [3,4]. In this paper, we consider color and texture as two sufficient and uncorrelated feature representations of an image. Inspired by SVM
25、Act ive, we proposed a novel active learning method, Co-SVM. Firstly, SVM classifiers are separately learnt in different feature representations and then these classifiers are used to cooperatively select the most informative samples from the unlabeled data. Finally, the informativesamples are retur
26、ned to users to ask for labeling. 2. Support vector machines Being an effective binary classifier, Support Vector Machines (SVM) is particularly fit for the classification task in relevance feedback of image retrieval [5]. With the labeled images, SVM learns a boundary (i.e., hyper plane) separati
27、ng the relevant images from the irrelevant images with maximum margin. The images on a side of boundary areconsidered as relevance, and on the other side are looked as irrelevance. Given a set of labeled images (x1, y1), . . . , (xn, yn), xi is the feature representation of one image, yi ∈ {?1,+1}
28、is the class label (?1 denotes negative and +1 denotes positive). Training SVM classifier leads to the following quadratic optimization problem: S.t.: where C is a constant and k is the kernel function. The boundary (hyper plane) is Where are any support vectors satisfied: The classificati
29、on function can be written as 3. Co-SVM 3.1. Two-view scheme It is natural and reasonable to assume that color features and texture features are two sufficient and uncorrelated views of an image. Assume that x = {c1, . . . , ci, t1, . . . , tj } is the feature representation of an image, where {
30、c1, . . . , ci } and {t1, . . . , tj } are color attributes and texture attributes, respectively. For simplicity, we define the feature representation space V = VC VT , and {c1, . . . , ci} ∈ VC, {t1, . . . , tj} ∈ VT . In order to find relevant images as much as possible, like the general relevan
31、ce feedback methods, SVM is used to learn a classifier h on these labeled samples with the combined view V at the first stage. The unlabeled set is classified into positive and negative by h. Then m positive images are returned to user to label. At the second stage, SVM is used to separately learn t
32、wo classifiers hC and hT on the labeled samples only with color view VC and texture view VT , respectively. A set of unlabeled samples that disagree between the two classifiers is recommended to user to label, which named contention samples. That is, the contention samples are classified as positive
33、 by hC (CP) while are classified as negative by hT (TN), or are classified as negative by hC (CN) while are classified as positiveby hT (TP). For each classifier, the distance between sample and the hyper plane (boundary) can be looked as the confidence degree. The larger the distance, the higher th
34、e confidence degree is. In order to ensure that users can label the most informative samples, the samples which are close to hyper plane in both views are recommended to user to label. 3.2. Multi-view scheme The proposed algorithm in two-view case is easily extended to multi-view scheme. Assume t
35、hat the feature representation of a color image is defined as V = V1 V2 Vk, k>2, each Vi, i = 1, . . . , k corresponds to a different view of the color image. Then k SVM classifiers hi can be individually learnt on each view. All unlabeled data are classified as positive (+1) or negative (?1) by
36、k SVM classifiers, respectively. Define the confidence degreeD(x) =______ki=1sign(hi(x))_____.The confidence degree can reflect the consistency of all classifiers on a specified example. The higher the confidence degree, the more consistent the classification is. Inversely, lower degree indicates th
37、at the classification is uncertain. The labeling on these uncertain samples will result in maximum improvement of performance. Therefore, the unlabeled samples whose confidence degrees are the lowest are considered as the contention samples. 3.3. About SVM SVM (Support Vector machine, support ve
38、ctor machine) method [4] is based on statistical learning theory and the theory of VC dimension based on structural risk minimization principle, according to the limited sample information in the model complexity and learning ability of the most sought between good compromise to obtain the best gene
39、ralization ability. The main idea of SVM is a hyperplane as the decision surface, making the positive examples and counterexamples of separation between the edges is maximized. For the two-dimensional linear separable case, so H to the two types of training samples is not wrong to separate classi
40、fication line, H1, H2, respectively from the classification of various types in the sample line and the recent classification of lines parallel to the line, they shall called the interval distance between categories. The so-called optimal separating line is to ask the correct classification of line
41、not only be able to separate the two, but also the largest classification interval. In high dimensional space, the optimal classification line has become the optimal classification surface. 4. Experiments To validate the effectiveness of the proposed algorithm in improvement of performance, we com
42、pare it with Tong & Chang’s SVMAct ive and the traditional relevance feedback algorithm using SVM. Experiments are performed on a subset selected from the Corel image CDs. There are 50 categories in our subset. Each category contains 100 images, 5000 images in all. The categories have different sema
43、ntic meanings, such as animal, building, landscape, etc. In our experiments, the main purpose is to verify if the learning mechanisms of Co-SVM are useful, so we only employed simple color and texture features to represent images. The color features include 125-dimensional color histogram vector a
44、nd 6-dimensional color moment vector in RGB. The texture features are extracted using 3-level discrete wavelet transformation (DWT). The mean and variance averaging on each of 10 subbands are arranged to a 20-dimensional texture feature vector. RBF kernel is adopted in SVM classifiers. The kernel wi
45、dth is learnt by cross-validation approach. The first 10 images of each category, 500 images in total,are selected as query images to probe the retrieval performance. In each round, only the top 10 images are labeled and 10 least confident images selected from contention set are labeled. All accura
46、cy in the following text is the averaging accuracy of all test images. Figs. 2 and 3 are the accuracy vs. scope curve of the three algorithms after the third and fifth rounds of relevance feedback, respectively. From the comparison results we can see that the proposed algorithm (Co-SVM) is better th
47、an SVMAct ive (active SVM) and the traditional relevance feedback method (SVM). Furthermore, we investigate the accuracy of various algorithms within top 10 to top 100, and with five rounds of feedback. For limited space, we only picture the results of top 30 and top 50 in Figs.1and 5, respectively.
48、 The detailed results are summarized in Table 1. The results depicted in Table 1 show thatCo-SVM achieves the highest performance. 5. Related works Co-training [3] and co-testing [4] are two representative multi-view learning algorithms. Co-training algorithm adopts cooperative learning strategy a
49、nd requires that the two views of data are compatible and redundant. We have attempted to augment the performance of both color and texture classifiers by combining co-training, but the results were worse. Considering the condition of co-training, it is not surprising to find that color attribute an
50、d texture attribute are not compatible but uncorrelated for a color image. In contrast, co-testing requires that the views should be sufficient and uncorrelated which makes the classifiers more independent for classification. Tong and Chang firstly introduced active learning approach to relevance f
51、eedback of image retrieval, SVMAct ive [2]. They think that the samples lying beside the boundary can reduce the version space as fast as possible, i.e. eliminating the hypotheses. Therefore, in each round of relevance feedback, the images that are closest to the hyperplane are returned to users for
52、 labeling. SVMAct ive is optimal for minimizing the version space in case of single view. The proposed algorithm can be regarded as an extension of SVMAct ive in multiple view case. 6. Conclusions In this paper, we proposed a novel active learning algorithm for selective sampling in relevance feed
53、back, Co-SVM. In order to improve the performance, the relevance feedback is divided into two stages. At the first stage, we rank the unlabeled images by their similarity to the query and let users to label the top images like the common relevance feedback algorithms. In order to reduce the labelin
54、g requirement, only a set of the most informative samples are selected by Co-SVM to label at the second stage. The experimental results show that the Co-SVM achieves obvious improvement compared with SVMAct ive and the traditional relevance feedback algorithm without active learning. Acknowledgemen
55、ts The first author was supported under Nokia Postdoctoral Fellowship. References [1] Y. Rui, T.S. Huang, S.F. Chang, Image retrieval: current techniques, promising directions and open issues, J. Visual Commun. Image Representation 10 (1999) 39–62. [2] S. Tong, E. Chang, Support vector machin
56、e active learning for image retrieval, in: Proceedings of the Ninth ACM International Conference on Multimedia, 2001, pp. 107–118. [3] A. Blum, T. Mitchell, Combining labeled and unlabeled data with co-training, in: Proceedings of the 11th Annual Conference on Computational Learning Theory, 1998, pp. 92–100. [4] I. Muslea, S. Minton, C.A. Knoblock, Selective sampling with redundant views, in: Proceedings of the 17th National Conference on Artificial Intelligence, 2000, pp. 621–626. [5] V. Vapnik, Statistical Learning Theory, Wiley, New York, 1998.
- 溫馨提示:
1: 本站所有資源如無特殊說明,都需要本地電腦安裝OFFICE2007和PDF閱讀器。圖紙軟件為CAD,CAXA,PROE,UG,SolidWorks等.壓縮文件請下載最新的WinRAR軟件解壓。
2: 本站的文檔不包含任何第三方提供的附件圖紙等,如果需要附件,請聯(lián)系上傳者。文件的所有權(quán)益歸上傳用戶所有。
3.本站RAR壓縮包中若帶圖紙,網(wǎng)頁內(nèi)容里面會有圖紙預(yù)覽,若沒有圖紙預(yù)覽就沒有圖紙。
4. 未經(jīng)權(quán)益所有人同意不得將文件中的內(nèi)容挪作商業(yè)或盈利用途。
5. 裝配圖網(wǎng)僅提供信息存儲空間,僅對用戶上傳內(nèi)容的表現(xiàn)方式做保護處理,對用戶上傳分享的文檔內(nèi)容本身不做任何修改或編輯,并不能對任何下載內(nèi)容負責(zé)。
6. 下載文件中如有侵權(quán)或不適當(dāng)內(nèi)容,請與我們聯(lián)系,我們立即糾正。
7. 本站不保證下載資源的準確性、安全性和完整性, 同時也不承擔(dān)用戶因使用這些下載資源對自己和他人造成任何形式的傷害或損失。
最新文檔
- 6.煤礦安全生產(chǎn)科普知識競賽題含答案
- 2.煤礦爆破工技能鑒定試題含答案
- 3.爆破工培訓(xùn)考試試題含答案
- 2.煤礦安全監(jiān)察人員模擬考試題庫試卷含答案
- 3.金屬非金屬礦山安全管理人員(地下礦山)安全生產(chǎn)模擬考試題庫試卷含答案
- 4.煤礦特種作業(yè)人員井下電鉗工模擬考試題庫試卷含答案
- 1 煤礦安全生產(chǎn)及管理知識測試題庫及答案
- 2 各種煤礦安全考試試題含答案
- 1 煤礦安全檢查考試題
- 1 井下放炮員練習(xí)題含答案
- 2煤礦安全監(jiān)測工種技術(shù)比武題庫含解析
- 1 礦山應(yīng)急救援安全知識競賽試題
- 1 礦井泵工考試練習(xí)題含答案
- 2煤礦爆破工考試復(fù)習(xí)題含答案
- 1 各種煤礦安全考試試題含答案